Gabriela Gutierrez, Head of Finance at Teads, gave this talk at the FP&A Summit.
Before I talk about the future of FP&A, I want to start from the very beginning. Why does FP&A even exist?
In my opinion, the main role of FP&A is to help businesses make better decisions. But how can we make better decisions?
We should start by looking at our responsibilities.
We’re in charge of the long-term planning. We monitor market trends, we understand in-depth business performance, and based on that, we provide insights and recommendations for future action. Overall, we’re essential in shaping the company's long-term strategy.
In this article, we'll look at:
- How FP&A improves decision-making
- The importance of making accurate financial predictions
- What can we achieve with machine learning?
- Case study: Achieving precision in budget forecasting
- Innovative forecasting using Python and Prophet
- Machine learning can't replace human judgment
- The future of FP&A is both human and AI-driven
How FP&A improves decision-making
I created a framework that figures out how we can make better decisions. The most important part is collecting the data. We can collect any type of business data and based on that, we create reports on variance analysis, and then we make predictions.
Predictions would involve us creating budgets and monthly forecasts, and then sharing those insights with different stakeholders.
And then we have the business partnering. We talk to the heads of different departments, decide on strategy, and then decide on budget.
That's how we make decisions. That strategy or budget will influence the future and those will become our actuals. And then we’ll collect the data again.
It’s a circle that starts from data and then ends with the strategy, but the strategy will change our future data.
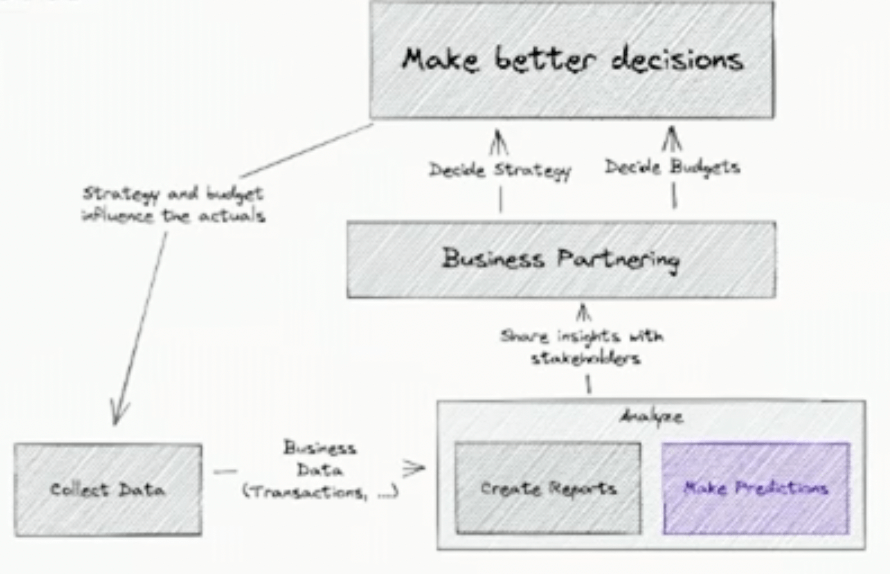
The importance of making accurate financial predictions
Now, I want to focus on how we make predictions and why predictions are so important.
Data holds insights that enable us to make better decisions and take different actions.
50 years ago, predictions were made based on human judgment. Professionals relied on their intuition and the experience that they developed over time. They had that gut feeling about what a prediction would look like.
The problem is that we sacrifice accuracy. We use information to inform our God and then we trust it.
To make predictions today, we collect data and include different departments in our company like the IT or analytical department. They collect the data and then they share it with us.
But what we take from that data is a summary. Otherwise, we wouldn't be able to comprehend it. We have thousands or millions of rows of data that our human brain won't be able to comprehend without having a summary.
Summaries are processed by humans and then most of the time are used on a spreadsheet, dashboard, or any analytical application.
But we should be challenging if those summaries in a spreadsheet are really the best solution.
Human judgment is still the main driver, and that usually wouldn't be a problem. But how can we make decisions around data? How can we decide which data to choose from to create our forecasts or budgets from this big pool of data? Which data should we exclude? How can we even make that decision? What's the framework?
Data isn’t enough to isolate us from human bias.
A spreadsheet can’t scale with the data. It has limited statistical methods, it's inaccurate, it sometimes involves a lot of manual work, it's time-consuming to maintain these models, and it's very difficult to create different scenarios.
That's why I say, let's forget about spreadsheets for now and give machine learning a chance.
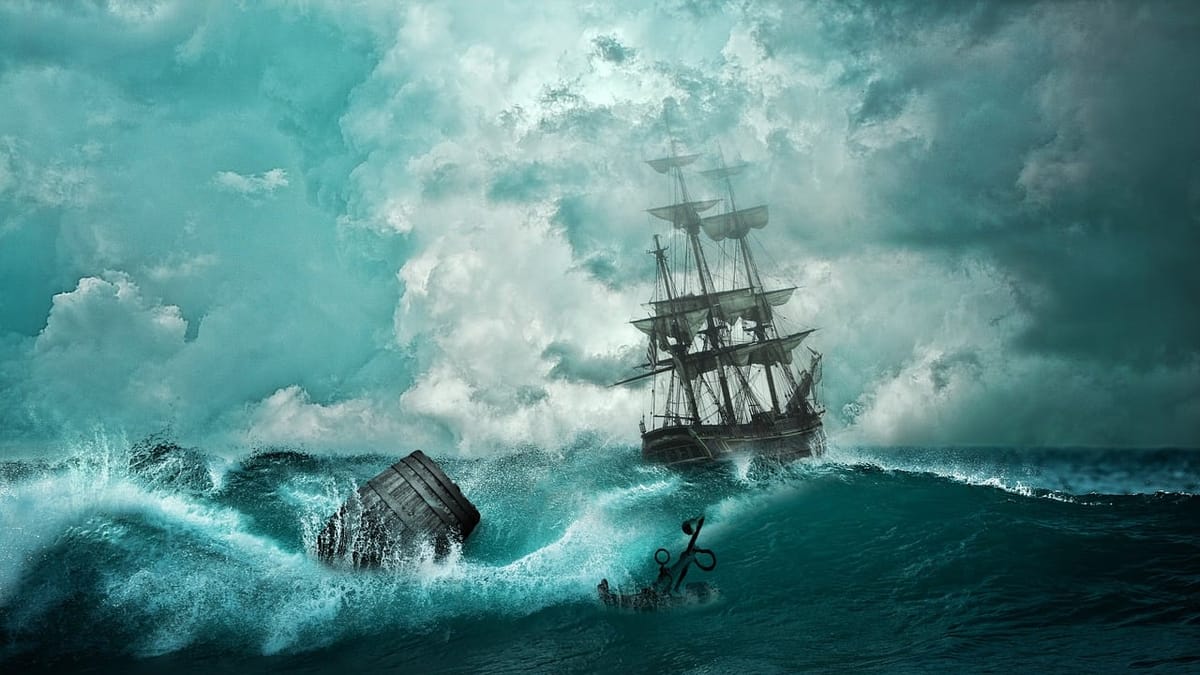
What can we achieve with machine learning?
So, why machine learning?
Machine learning can scale, it can take in thousands or millions of rows, accuracy, analyze data faster, reduce human bias, adapt to unknown situations, and plan for complex scenarios.
Machine learning can be trained, and it’ll know exactly which data to choose and which data to ignore. Machine learning doesn't have a problem with scaling, so we can just provide it with all the information we want and it’ll give us information that we can't understand.
I like this quote:
“Machine learning creates value by improving the quality of decisions.”
Ajay K. Agrawal, Avi Goldfarb, Joshua Gans
FP&A’s main role is to improve the decision-making process, and machine learning and FP&A can be friends; they complement each other. We have a forecasting problem and machine learning can help us to solve that problem.
Case study: Achieving precision in budget forecasting
In my previous role, we had tons of data and we weren't able to scale it. We were forecasting our budget and monthly forecasts on a spreadsheet, and the problem was that we weren’t even able to use the relevant data, so we needed to find a solution.
The solution was to start using machine learning algorithms. I'd found an open-source algorithm called Prophet, which was developed by Facebook to forecast traffic.
Once I’d implemented that in the case study that I was able to develop, the budget delta was less than 1% in a year. It was fully automated. The profitability increased by 62% for one product, and the team was able to focus on business partnering and guiding the strategy.
Once we knew that our forecast was accurate, we could see what the future would look like and we could take action.
The variables that we took were different. We took internal variables such as visits, page views, and revenue. We also took external variables like the inflation rate and the COVID stringency index. We took the holidays because for us, every time there was a bank holiday, our visits would increase. Every time it rained, we’d increase it for a week or a month. Our revenues would quadruple.
So, those are the types of data that we can include in our budget to see how much it will impact revenue and cost.
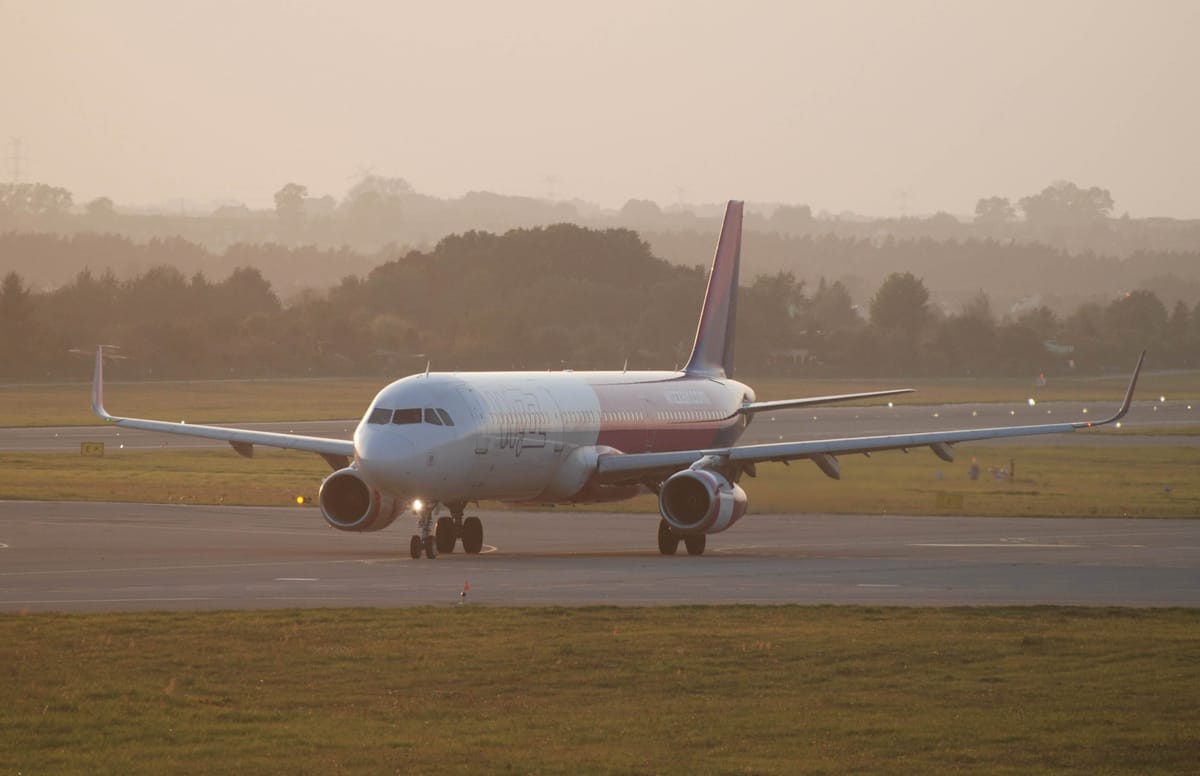
Innovative forecasting using Python and Prophet
I was still using a spreadsheet, but only as a place to load and store data, not for analysis. It was a workflow of loading the data to a Python Prophet model. Then once I ran the model I restored it back into a spreadsheet. The challenge was that not everyone in the team was able to code in Python, so we needed to find a mediator for us to run it but also share it, so we’d increase transparency within the team.
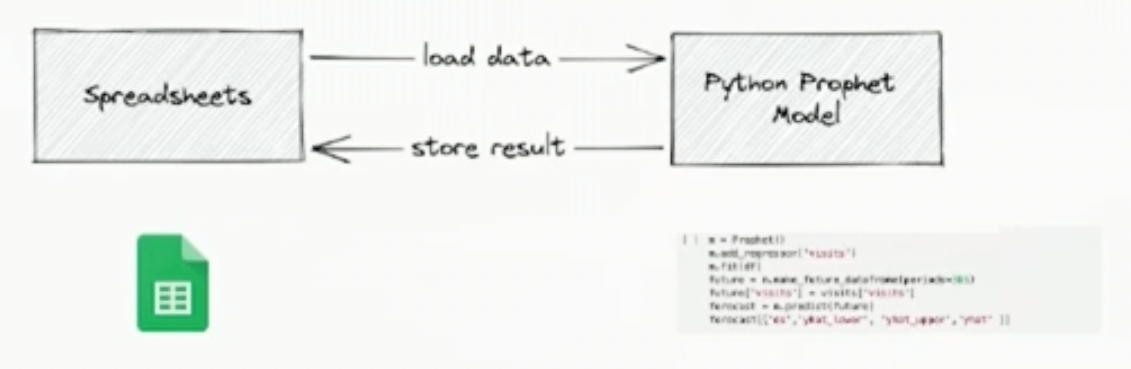
So, let me tell you how I did it.
I took sales data from 1992 until 2019. I told the model we had this data that I wanted to forecast for the next future periods. Then I told it that it was on a monthly basis, and it gave me all the information directly. It took three seconds to run it and then I had a new forecast.
It tells me when the data has a different trend, so I can go back and we can take a look at what happened in specific time periods.
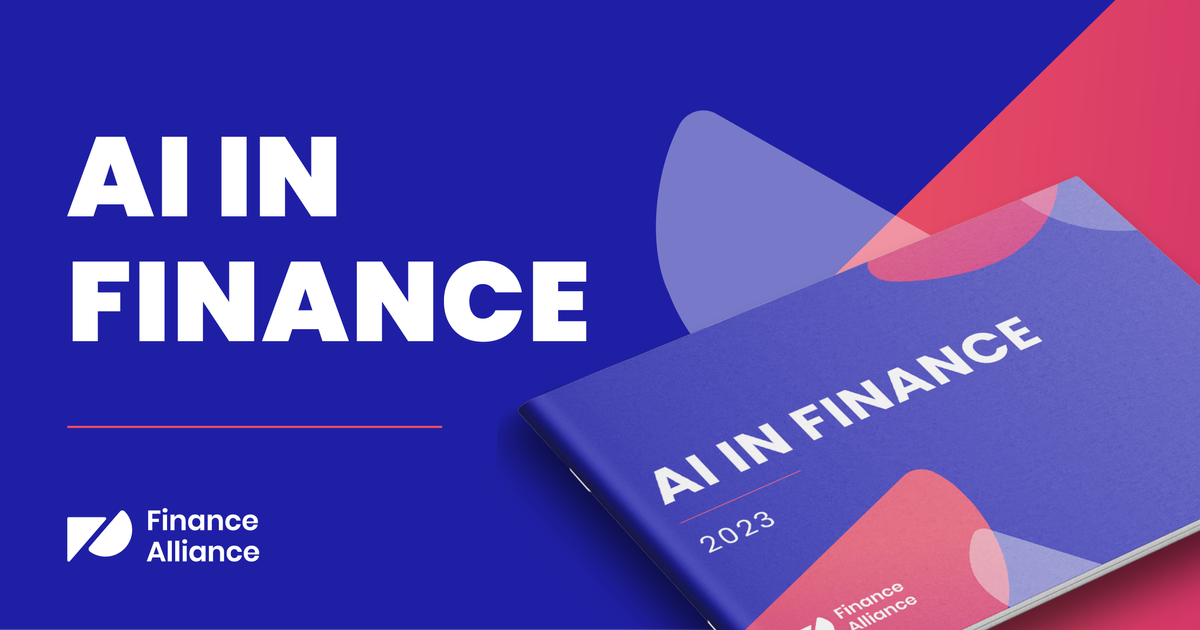
Machine learning can’t replace human judgment
So, are the machines replacing us? Not really.
The missing part of machine learning is the human judgment. Human judgment will become more valuable over time because machines will become better at forecasting, but they don't know what's happening in the business and they won't be able to understand it.
FP&A are the ones who can identify things like when we’re introducing a new line of products, and actually, this product isn’t performing because of sales.
Our judgment will inform what the focus will look like and the ones that are still in the loop.
So, what does human judgment look like in practice? I’ll give you another example.
We were so accurate in our forecast that we already knew what our revenues would look like for the next 24 months, but it still wasn’t within the company’s goals. We already knew that we had $100 million, and we needed $200 million. So what could we do now to get to that $200 million?
That's when we started to introduce dynamic pricing.
We either needed to adapt and then succeed to the $200 million, or we would be in stagnation. Or, in other cases, the company could decline.
Without predictions, we wouldn't have been able to achieve this.
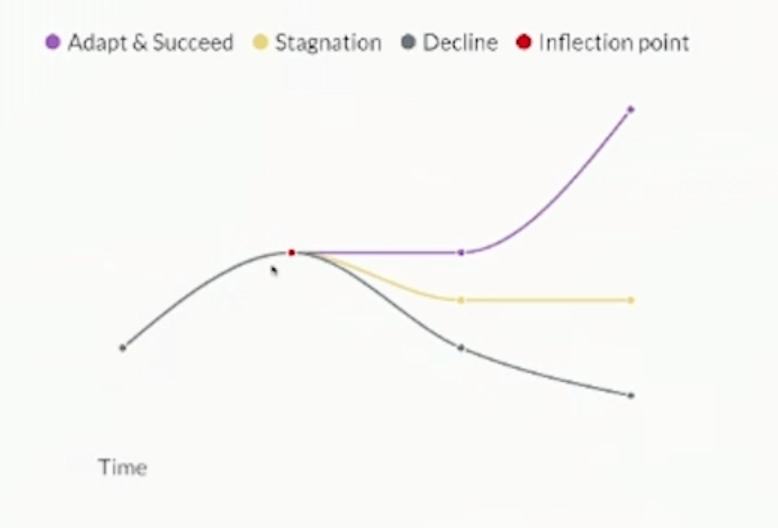
The future of FP&A is both human and AI-driven
In summary:
- The human-driven predictions mean that we have a cognitive bias.
- In FP&A, we’re data-driven but we still don't leverage all the data.
- AI is not the optimal solution because humans aren’t in the loop.
- Both human and AI-driven is the optimal solution.
But what's next? We’ll have more accurate predictions, we’ll be able to make better decisions, and then at some point, and if needed, our strategy will change.
Machine learning tools in FP&A are still quite new and more tools will be coming. But once we’re able to get there, we’ll be able to focus more of our time on becoming better business partners.
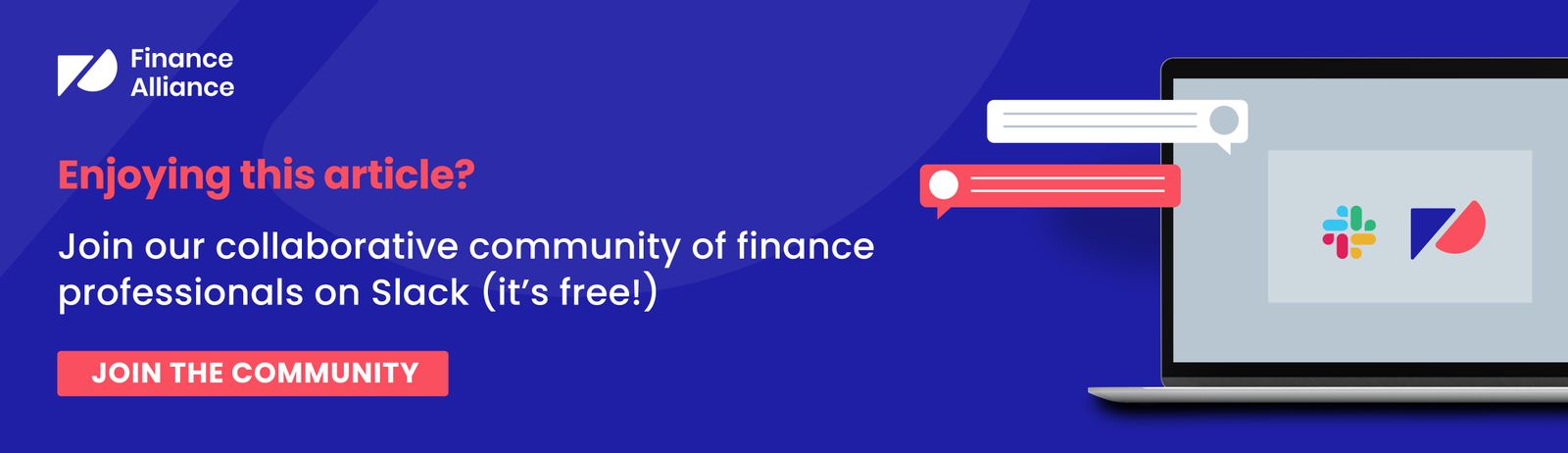